Optimizing Production Efficiency with Predictive Maintenance - A case study in the manufacturing industry
In the rapidly evolving world of manufacturing, efficiency remains a constant watchword. Businesses are continuously searching for new methods and strategies to boost efficiency because failure to do so will result in loss of revenue and wastage of resources. Equipment breakdowns and unplanned downtimes are major sources of these inefficiencies that harm productivity and revenue streams of manufacturing companies. With traditional reactive maintenance causing longer outage time and preventive maintenance being limited to detecting only surface-level issues, the top industry players are turning to predictive maintenance to maintain year-round efficiency at manufacturing plants.
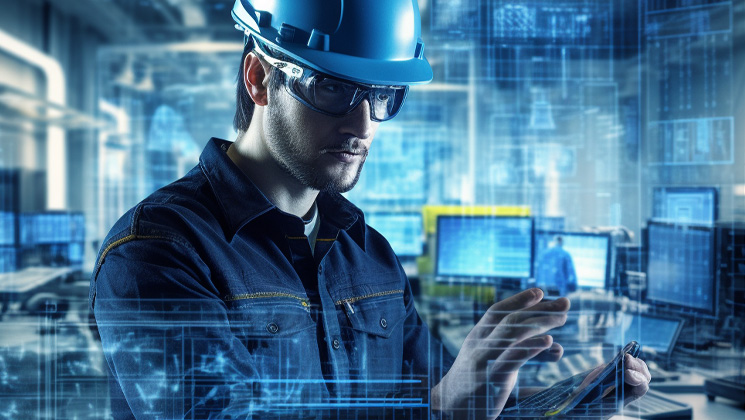
Predictive Maintenance: Overview, advantages and disadvantages
Predictive maintenance (PdM) is a data-driven approach that uses constant monitoring of the performance and condition of equipment to predict the best times to schedule maintenance activities. Predictive maintenance aims to estimate time-to-failure to enable early scheduling of corrective actions, thus maximizing equipment lifespan and reliability. A technique that is becoming increasingly sophisticated thanks to emerging technologies, predictive maintenance broadly involves the following components: data collection, processing and analysis, condition monitoring, time-to-failure prediction, and maintenance scheduling.
Adopting predictive maintenance comes with several advantages, the first being boosting production efficiency. According to McKinsey and Company, PdM leads to a 20% increase in maintenance efficiency. Also, it reduces unplanned downtimes, which cost up to $260,000 per hour, according to Aberdeen Group . Hence, it ensures cost savings incurred during rule-based maintenance at regular intervals while assuring the maximization of asset lifetime value. The aggregate effect is an increase in profitability and revenue generation for the business.
An argument against predictive maintenance is, perhaps, the complexity associated with the implementation process. A fully functional PdM program requires a highly skilled team to ease the transition from the previous system to the advanced software and hardware needed for condition monitoring and data analytics. However, this process can be simplified with strategic planning that divides the implementation program into phases for easier execution.
Role of data analytics in predictive maintenance
Data is central to predictive maintenance, and the data types typically include sensor data, historical maintenance records, and environmental data. Technologies such as acoustic, vibration analysis, infrared thermography, sound level measurements, and corona detection generate sensor data that tell experts about the general conditions of the machines. Historical maintenance records provide insights like past failure analysis, equipment lifespan and performance trends, and maintenance history for a more rounded perspective. Lastly, environmental data monitor external conditions - temperature, humidity, air quality - that may affect equipment functioning.
Data analytics is the next step after collecting the sensor, historical, and environmental data. There are two main methods of analysing data in a PdM program: statistical analysis and artificial intelligence.
Statistical analysis uses mathematical models to process data and make conclusions. Subsequently, rule-based models are developed for pre-empting equipment failure. For example, the analysis may reveal that a machine fails at certain temperatures, prompting personnel to create a rule-based system that triggers an alarm before reaching that temperature point.
However, making predictions is a significant limitation of statistical analysis. Also, it is often difficult considering data in the broader context as these methods typically consider different parameters in isolation.
Artificial intelligence and machine learning offset those limitations by introducing robust algorithms that learn from data and identify patterns most human analysts would miss using techniques such as support vector machines and neural networks. Hence, it can be used for making predictions with a high degree of certainty. Moreover, unlike statistical models which consider parameters in isolation, machine learning uses a more comprehensive approach that considers all inputs simultaneously. Logically, machine learning is now at the forefront of data analytics in predictive maintenance, and it continues to produce exciting returns for companies implementing it.
5 Challenges manufacturers may face when implementing predictive maintenance
Although the end results are worthwhile, the process of implementing predictive maintenance can be challenging. It is important for manufacturing companies planning to shift their preferred means of maintenance to a predictive method to be well aware of what it entails to launch and sustain a successful program.
Here are 5 biggest challenges to anticipate as you consider adopting predictive maintenance in your company:
- Data Quality
The difference between the success and failure of a PdM program is the quality of data made available. High-quality and accurate data is necessary for the program to meet its goals, so businesses must put in much work to validate all data sources from the onset.
- Data Integration and Standardisation
It is important to collate data from independent sources and then standardize the information to create a singular view of the equipment’s condition. The processes involved are often complex and time-consuming as the data will likely be scattered across numerous sources and exist in various formats and structures.
- Cost
Launching a predictive maintenance system requires an investment of high upfront costs. The manufacturing companies will have to pay for hardware and software necessary for data collection and processing. Additional funds must also be reserved for the personnel to supervise the program.
- Technical Expertise
Every predictive maintenance program needs the presence of experts such as data analysts, data scientists, and machine learning experts. Such expertise is in high demand and short supply, making hiring them quite challenging.
- Adoption
Moving from reactive or preventive maintenance to PdM is a significant change for a company and its personnel. Consequently, some stakeholders may be reluctant to adopt this new maintenance process, considerably slowing down the implementation process.
Practice implementation – a manufacturing case study
Numerous manufacturing companies have implemented a predictive maintenance program and achieved remarkable results. A good example is Duke Energy, one of the largest energy companies in the United States. Following a catastrophic failure that cost $10 million in fines and a significant loss of revenue, the company launched its SmartGen program. The SmartGen initiative relied on the Industrial Internet of Things (IIoT) and big data analytics for condition monitoring and predictive maintenance. The program was successful and Duke Energy saved $31.5 million in repair costs within the first three years.
Similarly, BMW Group implemented a PdM program, utilizing sensors, data analytics, and artificial intelligence to design a system for maintaining their machines. The applications of BMW’s system are diverse. For example, it is used in vehicle assembly to prevent downtime in conveyor belts and in body shops to ensure the welding guns stay functional. Visual warnings and alerts are sent to employees to inform them when equipment maintenance is due. BMW’s program is made even more sustainable due to their cloud-based platform which allows seamless data exchange within the entire global BMW Group network. Because they execute predictive maintenance at such a prominent level, BMW can now maintain production efficiency and ensure profitability . These case studies are only two of many that illustrate the value of predictive maintenance in the manufacturing industry.
The Future of predictive maintenance and data analytics
In this age of Industry 4.0 technologies such as artificial intelligence, machine learning, Industrial Internet of Things (IIoT), big data analytics, and automation, the future of predictive maintenance is quite promising. According to The World Economic Forum, adopting these technologies will lead to a 15-20% increase in global manufacturing productivity by 2030.
AI and machine learning are capable of advanced predictive analytics that will improve accuracy and scalability for businesses. IIoT provides seamless interconnectivity between hardware and software, ensuring rapid data exchange between machines and human operators. Therefore, this means PdM can be implemented at a higher level than ever before, enhancing its profitability for manufacturers. Finally, the emergence of cloud computing means manufacturers can continue collecting more data for analysis without the limitation of low storage capabilities.
Translate business strategy into actionable insights
Minimizing downtime and maximizing the lifespan of equipment is key to optimizing production efficiency and revenue generation. Predictive maintenance is a strategy that provides comprehensive solutions to the challenges manufacturers face. It’s a data-intensive approach, but the availability of artificial intelligence, cloud computing, and Industrial Internet of Things (IIoT) remarkably simplify the process for companies looking to implement this solution.
At ACTUM Digital, we provide the final piece of the puzzle by helping your business introduce these new technologies and design effective data strategies for your PdM program to thrive. Find out how we can help you reach your production efficiency targets faster and more cost-effectively here.
You may also like...
[19/05/2023] How Big Data Analytics Impacts Manufacturing & Supply Chain
Unleash endless possibilities in manufacturing supply chain operations with big data analytics. Discover implementation steps for maximum benefits.
Read the Insight